The landscape of SVOD (subscription video on demand) is experiencing significant growth. According to Digital TV Research, global SVOD subscriptions are projected to increase by 321 million between 2023 and 2029, reaching a total of 1.79 billion. This data highlights the continued expansion and popularity of SVOD services making it the principal source of OTT revenue, projected to grow by $30 billion between 2023 and 2029, culminating in a total of $139 billion. Additionally, AVOD (advertising-based video on demand) is also set to see substantial growth. AVOD revenues are projected to reach $68 billion by 2029, an increase of $30 billion from $39 billion in 2023. This surge reflects the increasing importance and monetisation potential of ad-supported streaming content. (Digital TV Research)
Problem Statement
Our client, a leading global OTT service provider wanted to predict new subscriber acquisitions driven by a new season of a show. For example, if Season 2 of Show "X" is launching next month, they wanted to predict the total acquisitions during its airing period. They needed an accurate forecasting solution.
At Kantar, we proposed an AI-powered solution to meet their prediction needs and establish a data-driven baseline for benchmarking show and season acquisitions. This initiative has revolutionised their marketing, content strategy, and financial forecasting.
Challenges
1. FTS to show attribution: Accurately attributing free trial sign-ups (FTS) to specific shows to understand which content drives acquisitions was a challenge.
2. Data sparsity: Sparse data at the show-season level, especially for shows with single seasons or no simulcast data, posed a challenge for building robust predictive models.
3. Limited data points: Unavailability of enough historical data points at the show-season level for training machine learning models.
4. Data diversity: Integrating and cleaning vast amounts of data from various sources, including subscription data, viewership patterns, and show metadata from external sources posed a challenge.
Designed Solution and Methodology
Kantar proposed a multi-step approach to assist the client with predictions and address the challenges.
Multi-Step Approach:
1. Data collection and integration: Integrated data from multiple sources, e.g. subscription and viewership data, along with show metadata from external sources.
- Also identified key features influencing acquisitions, including:
- Previous season performance metrics like previous season’s FTS, views, SVOD users
- Platform performance metrics like average FTS of recent months, current show perception and penetration percentage
- Show metadata like previous season ratings, script rating, genre, popularity, release timing and number of dubbed episodes
- Calendar and seasonal variables like pandemic indicator, holiday indicator, event flag
2. FTS to show attribution: Explored multiple attribution methodologies such as First Touch, Last Touch and Custom Rule-based Attribution. After careful assessment and considering client preferences, we selected First Touch Attribution, where the first show watched by the user in the FTS period is attributed to the subscription.
3. Predictive model evaluation: Evaluated various machine learning algorithms to identify the best model with the highest accuracy through extensive cross-validation techniques. Some of the algorithms considered include:
- Seemingly unrelated regression (SUR): To handle interdependencies among the different shows.
- Mixed effects: To capture both combined platform and similar show behaviour (fixed effects) and show specific effects (random effects) within the data.
- Ordinary least squares (OLS): For its simplicity and interpretability.
- LASSO regression: For effective feature selection and regularisation.
- XGBoost: For its robustness and superior performance in prediction.
Each model was rigorously tested to ensure robustness and reliability. Model selection was primarily based on MAPE (mean absolute percentage error) and weighted MAPE, which provided a nuanced evaluation of model accuracy, especially in the presence of diverse data points with varying importance.
4. Model training and validation: Split the data into training and validation sets to ensure model robustness. Used LOSO (leave one season out) cross-validation technique to evaluate model performance. A staggering accuracy of >85% was attained.
5. Model implementation: Developed an ensemble of XGBoost and Mixed Effects Model, which captured both show-level trends (random effects) and collective behaviour across the platform and similar shows (fixed effects), allowing for more accurate and granular predictions.
6. Dashboard development: Developed interactive dashboards to visualise predictions and the attained actuals, allowing stakeholders to get a detailed view of the acquisition performance of the respective shows and seasons.
Platform and Technologies Used
Solution Flow
Our AI-powered acquisition potential predictor has revolutionised the client's approach, delivering:
1. Data-driven decisions & efficiency: Replaced manual predictions with a semi-automated AI solution, enabling data-backed content investment and marketing strategies, streamlined workflows, and reduced analysis time.
2. Benchmarking: Established a data-driven baseline for show and show-season acquisitions, improving resource allocation and decision-making, potentially adding over $4 million in revenue.
3. Optimising marketing spend: Improved marketing ROI for content-specific campaigns, boosting ROI by over 15% for premium shows and generating additional millions in revenue.
4. Financial forecasting: Enabled accurate prediction of subscriptions driven by new seasons, aiding in revenue forecasting and financial planning. This contributed to a 3.1% increase in active subscription base, resulting in a 3.3% incremental revenue boost.
5. Content investment decisions: Helped the client decide which shows to invest in by predicting their potential to drive new acquisitions, supporting multi-million dollar decisions.
6. Release strategy optimisation: Helped determine the optimal timing for new season releases to maximise user engagement and acquisitions, leading to a 3% increase in views.
The success of this project can be extended to various other domains:
1. Predict performance of new products: Forecast the success of new product launches across retail, technology, and other industries.
2. Marketing campaign optimisation: Enhance effectiveness of marketing campaigns across various sectors.
3. Content acquisition & production: Media companies can leverage AI to prioritise content acquisition and production based on predicted audience engagement.
Problem Statement
Our client, a leading global OTT service provider wanted to predict new subscriber acquisitions driven by a new season of a show. For example, if Season 2 of Show "X" is launching next month, they wanted to predict the total acquisitions during its airing period. They needed an accurate forecasting solution.
At Kantar, we proposed an AI-powered solution to meet their prediction needs and establish a data-driven baseline for benchmarking show and season acquisitions. This initiative has revolutionised their marketing, content strategy, and financial forecasting.
Challenges
1. FTS to show attribution: Accurately attributing free trial sign-ups (FTS) to specific shows to understand which content drives acquisitions was a challenge.
2. Data sparsity: Sparse data at the show-season level, especially for shows with single seasons or no simulcast data, posed a challenge for building robust predictive models.
3. Limited data points: Unavailability of enough historical data points at the show-season level for training machine learning models.
4. Data diversity: Integrating and cleaning vast amounts of data from various sources, including subscription data, viewership patterns, and show metadata from external sources posed a challenge.
Designed Solution and Methodology
Kantar proposed a multi-step approach to assist the client with predictions and address the challenges.
Multi-Step Approach:
1. Data collection and integration: Integrated data from multiple sources, e.g. subscription and viewership data, along with show metadata from external sources.
- Also identified key features influencing acquisitions, including:
- Previous season performance metrics like previous season’s FTS, views, SVOD users
- Platform performance metrics like average FTS of recent months, current show perception and penetration percentage
- Show metadata like previous season ratings, script rating, genre, popularity, release timing and number of dubbed episodes
- Calendar and seasonal variables like pandemic indicator, holiday indicator, event flag
2. FTS to show attribution: Explored multiple attribution methodologies such as First Touch, Last Touch and Custom Rule-based Attribution. After careful assessment and considering client preferences, we selected First Touch Attribution, where the first show watched by the user in the FTS period is attributed to the subscription.
3. Predictive model evaluation: Evaluated various machine learning algorithms to identify the best model with the highest accuracy through extensive cross-validation techniques. Some of the algorithms considered include:
- Seemingly unrelated regression (SUR): To handle interdependencies among the different shows.
- Mixed effects: To capture both combined platform and similar show behaviour (fixed effects) and show specific effects (random effects) within the data.
- Ordinary least squares (OLS): For its simplicity and interpretability.
- LASSO regression: For effective feature selection and regularisation.
- XGBoost: For its robustness and superior performance in prediction.
Each model was rigorously tested to ensure robustness and reliability. Model selection was primarily based on MAPE (mean absolute percentage error) and weighted MAPE, which provided a nuanced evaluation of model accuracy, especially in the presence of diverse data points with varying importance.
4. Model training and validation: Split the data into training and validation sets to ensure model robustness. Used LOSO (leave one season out) cross-validation technique to evaluate model performance. A staggering accuracy of >85% was attained.
5. Model implementation: Developed an ensemble of XGBoost and Mixed Effects Model, which captured both show-level trends (random effects) and collective behaviour across the platform and similar shows (fixed effects), allowing for more accurate and granular predictions.
6. Dashboard development: Developed interactive dashboards to visualise predictions and the attained actuals, allowing stakeholders to get a detailed view of the acquisition performance of the respective shows and seasons.
Platform and Technologies Used
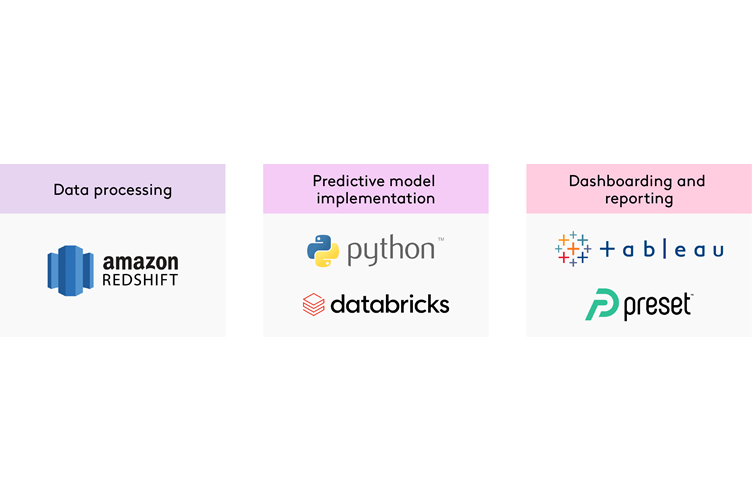
Solution Flow
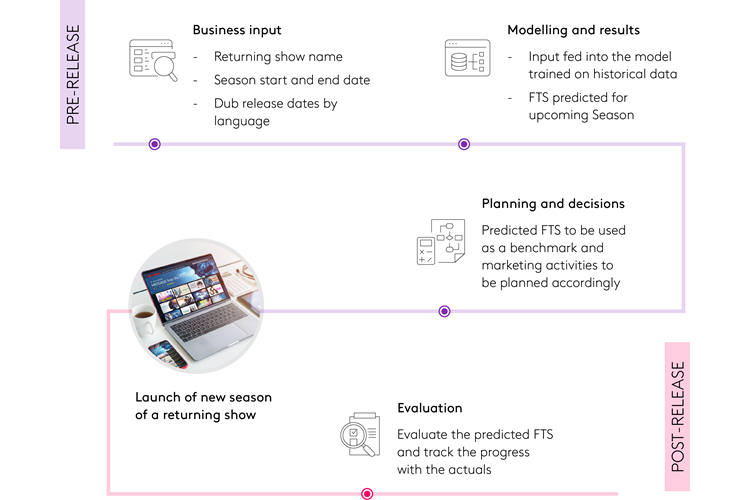
The Impact
Our AI-powered acquisition potential predictor has revolutionised the client's approach, delivering:
1. Data-driven decisions & efficiency: Replaced manual predictions with a semi-automated AI solution, enabling data-backed content investment and marketing strategies, streamlined workflows, and reduced analysis time.
2. Benchmarking: Established a data-driven baseline for show and show-season acquisitions, improving resource allocation and decision-making, potentially adding over $4 million in revenue.
3. Optimising marketing spend: Improved marketing ROI for content-specific campaigns, boosting ROI by over 15% for premium shows and generating additional millions in revenue.
4. Financial forecasting: Enabled accurate prediction of subscriptions driven by new seasons, aiding in revenue forecasting and financial planning. This contributed to a 3.1% increase in active subscription base, resulting in a 3.3% incremental revenue boost.
5. Content investment decisions: Helped the client decide which shows to invest in by predicting their potential to drive new acquisitions, supporting multi-million dollar decisions.
6. Release strategy optimisation: Helped determine the optimal timing for new season releases to maximise user engagement and acquisitions, leading to a 3% increase in views.
Possible Applications in OTT and Other Domains
The success of this project can be extended to various other domains:
1. Predict performance of new products: Forecast the success of new product launches across retail, technology, and other industries.
2. Marketing campaign optimisation: Enhance effectiveness of marketing campaigns across various sectors.
3. Content acquisition & production: Media companies can leverage AI to prioritise content acquisition and production based on predicted audience engagement.