Today, AI enables us to go further and faster for our clients, making strides that are redefining the relationship between business and consumers.
Thoughtfully and responsibly, we are now infusing GenAI into our core to reimagine the future better than anyone in the industry. Together, we’ll write the next chapter of brand history.”
Chris Jansen
CEO, Kantar
Kantar is your indispensable brand partner in a world being reimagined by AI
Innovation in AI is opening new frontiers in the collection, interpretation and application of human data. It enables even deeper understanding of how people everywhere think and act – and allows us to generate brand-defining insights at ever greater speed and scale.
You’ll find us innovating at the points in the marketing lifecycle where AI combined with the highest quality data and deep expertise can have the biggest growth impact.
We operate at the intersection of data science, marketing strategy and organisational performance to empower you with the most advanced AI capabilities that deliver growth for your brand and your team. Together we’ll harness the power of AI to shape your brand’s future.
BrandDynamics is a daily brand performance tracker to help you monitor your brand and competitors in real-time.
Supercharged with Kantar’s proprietary Trend AI technology to cancel the ‘noise’ in the data that distorts short-term metric movements and lets customers see category trends without time lag.
BrandHealth is a continuous brand health intelligence system that acts and reacts to threats and opportunities. Leveraging Kantar’s Trend AI and MDS framework supercharged with ML.
BrandStructures uses advanced analytics and ML to identify associations and help you focus on those that are likely to help grow your brand.
BrandDigital uses AI to analyse millions of search data points to anticipate trends in your category and predict who or what will likely cause disruption.
KaiA understands your brand growth needs and provides immediate answers to your questions in conversational, meaningful language. It connects Kantar intelligence to consumer marketing, brand, and sales data to give instant actionable insights that are easily understood and accessible anytime, anywhere.
Needscope AI decodes images, music, and videos based on their symbolic meaning, using a validated psychology-based framework. We help you differentiate your brand positioning and deliver a consistent experience across touchpoints.
GrowthFinderuses Kantar’s AI technology to segment by needs, attitudes, and behaviours to build a demand framework to tell the market's story. With GrowthFinder, you can put a value on each Demand Space segment in the framework to inform your portfolio strategy and investments. is a daily brand performance tracker to help you monitor your brand and competitors in real-time.
ConceptEvaluate AI leverages powerful, survey-trained AI to screen up to 100 early innovation concepts simultaneously, predicting in-market performance and unlocking new growth opportunities with unmatched speed and confidence.
TrendEvaluate harnesses AI-powered analysis of digital search data to uncover evolving consumer interests and intentions, helping you navigate shifting expectations and identify opportunities for meaningful innovations.
LINK AI reliably predicts an ad's in-market success in under 15 minutes without a consumer sample. Our advanced AI video processors extract every detail, from eye-catching visuals (pictures, objects, colours) to engaging audio (speech, sound effects) and even on-screen text. Then, state-of-the-art machine learning models analyse it all to predict your ad's effectiveness in record time.
LINK+ delivers razor-sharp insights tailored to your campaigns, whether you need a fast go/no-go call or in-depth guidance for early development— our powerful AI is behind our predictive key metrics for stronger ads that boost sales and brand value. Measure only what matters, optimise like a pro, and get results fast – all thanks to LINK+ unmatched flexibility.
LIFT ROI leverages Hamilton.AI to provide sound, unified metrics for marketing measurement and optimization. With intelligent automation, target a 10x-20x return on investment, guiding both strategic and tactical in-flight decisions across channels.
LIFT+ is a tech-driven optimisation tool that provides in-depth audience insights for your multichannel campaign.
Worldpanel Simulators use AI and ML to model and evaluate a range of 'what-if' scenarios to take the guesswork out of your major decisions.
By harnessing Kantar's unique behavioural data – from real shoppers over many years – we can help you identify which strategy is most likely to win.
Worldpanel Plus uses Shoppix, a proprietary smartphone app, where panellists can record their purchases and the motivations behind them in real-time. We use image processing and receipt decoding to join the dots on shopper behaviour across all trips, channels and industries - online and offline.
AI has been around in various forms for 50+ years, however Generative AI models such as ChatGPT have captured popular imagination in a way that previous technologies did not, and businesses all over the world are scrambling to create AI roadmaps and AI strategies that match the new pace of advances.
At Kantar, we have a rich history of innovation in this space, with AI and machine learning deeply embedded across our entire product suite and ways of working.
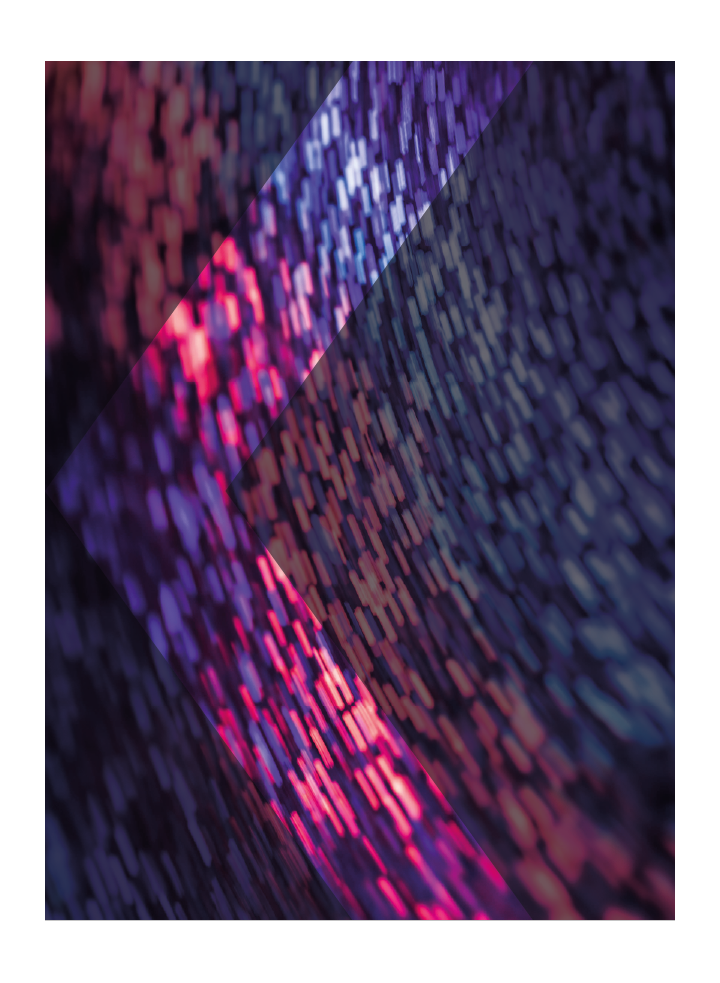
- Interpretable and trustworthy
- Human oversight
- Data management protocols
- Full impact assessments
- Close collaboration
- Embedded accountability
- People training and awareness
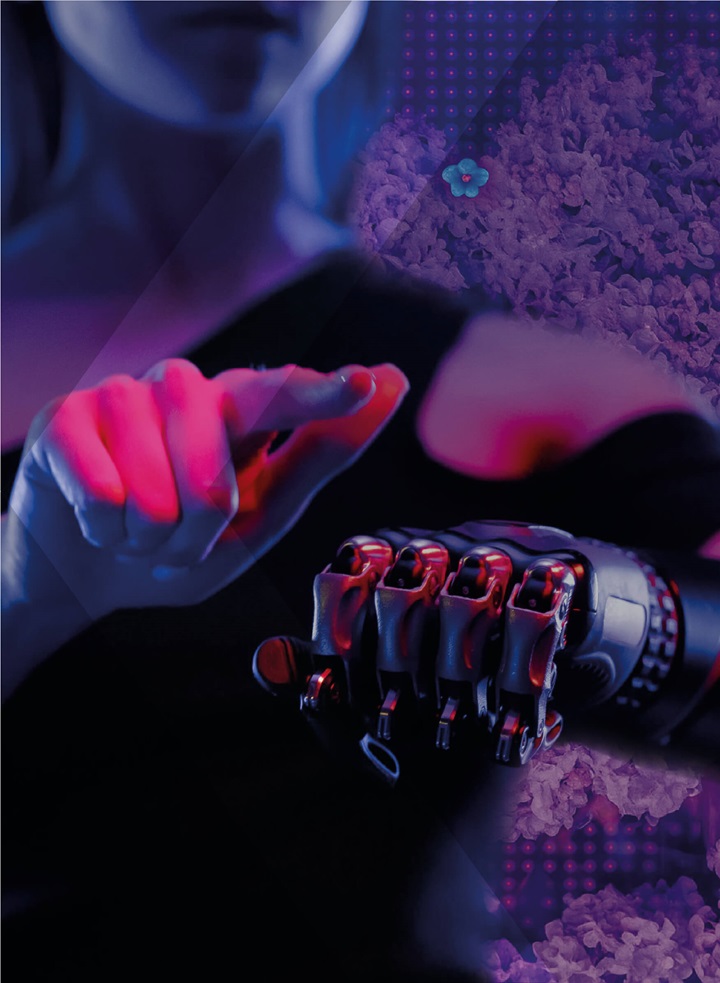
Apply our rigorous user permissions standards and ensure compliance with global data protection and privacy laws where PII/personal data is utilised or generated.
Quality
Ensure the data used for AI models is reliable, complete and relevant to research purposes.
Ownership and Use
Define and communicate the intellectual property rights involving the ownership of data, the AI Model, technologies, outcomes and other materials generated.
Lineage
Track the provenance of data and its transformation throughout the lifecycle.
Clients and 3rd parties
Assess whether our use impacts intellectual property rights belonging to clients or others.
Client Results
Kantar’s AI Lab
Explore how Kantar innovates with AI in collaboration with clients and partners.
Click here